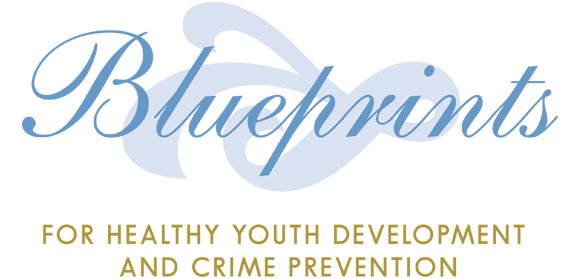
Small Schools of Choice
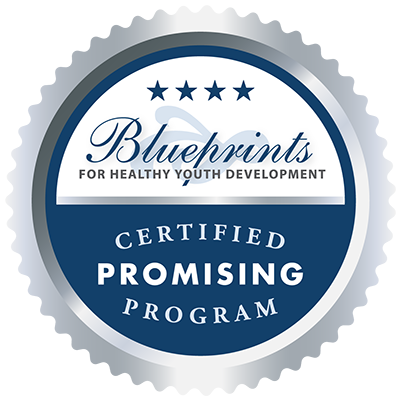
A high school reform model that is academically non-selective but provides academically rigorous instruction in small, personalized learning environments Along with smaller size, the model emphasizes real-world relevance and personalized relationships between teachers and students.
Program Outcomes
- Dropout/High School Graduation
Program Type
- School - Environmental Strategies
Program Setting
- School
Continuum of Intervention
- Universal Prevention
Age
- Late Adolescence (15-18) - High School
Gender
- Both
Race/Ethnicity
- All
Endorsements
Blueprints: Promising
Social Programs that Work:Near Top Tier
Program Information Contact
Rebecca Unterman
Senior Associate, K-12 Education
Manpower Demonstration Research Corporation (MDRC)
11965 Venice Blvd. #402
Los Angeles, CA 90066
Rebecca.Unterman@MDRC.org
Program Developer/Owner
Rebecca Unterman
Manpower Demonstration Research Corporation (MDRC)
Brief Description of the Program
New York City's Small Schools of Choice (SSCs) are academically non-selective but provide academically rigorous instruction in small, personalized learning environments for low-income high school students. Classes are generally smaller than those in public high schools, with more individualized attention given to students so that personal relationships are developed between teachers and students. In addition, the schools partner with outside organizations to assist with hiring exceptional teachers and staff as well as to provide students with opportunities to connect what they learn in school to real-world applications. Other implementations of the program such as in Chicago emphasize the small number of students, with schools having flexibility in choice of themes and curricula.
New York City's Small Schools of Choice (SSCs) are teacher, parent, and community-organized schools which are academically non-selective but designed to provide academically rigorous instruction in small, personalized learning environments for low-income students. Classes are generally smaller than those in public high schools, with more individualized attention given to students so that personal relationships are developed between teachers and students. In addition, the schools partner with outside organizations to assist with hiring exceptional teachers and staff as well as providing students with opportunities to connect what they learn in school to real-world applications.
In addition to small size and location mainly in disadvantaged neighborhoods, SSCs have other defining traits:
- All are created through a competitive proposal process in which teachers, parents, and community members come forward and form school planning committees.
- Most orient at least a portion of their school missions and curricula toward a specific academic, artistic, social justice, or professional theme.
- Most are founded in partnership with a local nonprofit organization or private employers that offer students learning opportunities in and out of the classroom, and additional support staff and resources.
- Almost all are provided additional philanthropic funds during their first four or five years of startup.
- The Department of Education offers exemptions from having to enroll special education students and English language learners during the two-year startup period.
- Almost all are started with the support of an intermediary school partner.
Outcomes
Primary Evidence Base for Certification
Study 1
Bloom and Unterman (2013) found that students enrolled in Small Schools of Choice in New York City were significantly more likely than similar students in other schools to
- Graduate high school
- Demonstrate college readiness in English.
Study 2
Abdulkadiroglu et al. (2013) found that students enrolled in Small Schools of Choice in New York City were significantly more likely than similar students in other schools to
- Perform better on Regents Exams in several fields
- Graduate high school on time
- Obtain more high school credits
- Stay on grade schedule
- Enroll in postsecondary education.
Brief Evaluation Methodology
Primary Evidence Base for Certification
Of the four studies Blueprints has reviewed, two studies (Studies 1 and 2) meet Blueprints evidentiary standards (specificity, evaluation quality, impact, dissemination readiness). The studies were conducted by independent evaluators.
Study 1
Bloom and Unterman (2013) used a lottery-based quasi-experimental design, with a lottery partially determining whether ninth-grade students would attend the small schools or standard high schools. The sample had 12,130 lottery students. The outcomes included test scores, graduation, and college attendance.
Study 2
Abdulkadiroglu et al. (2013) used a lottery-based quasi-experimental design, with a lottery partially determining whether ninth-grade students would attend the small schools or standard high schools. The sample had 10,204 lottery students. The outcomes included test scores, graduation, and college attendance.
Study 1
Bloom, H. S., & Unterman, R. (2013). Sustained progress: New findings about the effectiveness and operation of small public high schools of choice in New York City. New York: MDRC.
Unterman, R. (2014). Headed to college: The effects of New York City's small high schools of choice on postsecondary enrollment. New York: MDRC.
Study 2
Abdulkadiroglu, A., Hu, W., Pathak, P. A. (2013). Small high schools and student achievement: Lottery-based evidence from New York City. NBER Working Paper No. 19576.
Protective Factors
School: Opportunities for prosocial involvement in education
*
Risk/Protective Factor was significantly impacted by the program
Gender Specific Findings
- Male
- Female
Race/Ethnicity Specific Findings
- White
- Hispanic or Latino
- African American
Subgroup Analysis Details
Subgroup differences in program effects by race, ethnicity, or gender (coded in binary terms as male/female) or program effects for a sample of a specific racial, ethnic, or gender group:
- Study 1 (Bloom & Unterman, 2012, 2013) tested for subgroup differences in program effects by race, ethnicity, and gender and found equal benefits across subgroups. Tests for within-subgroup program effects found significant benefits for Blacks and Whites, Hispanics, and non-Hispanics, and males and females. In addition, Study 1 found subgroup effects by using a homogenous sample with 75% or more of students being eligible for free and reduced-price lunches.
- Study 2 (Abdulkadiroglu et al., 2013) tested for within-subgroup program effects and found significant benefits for African Americans, Hispanics, males, females, and economically disadvantaged students eligible for free and reduced-price lunches but did not report tests that compared the subgroups to one another.
Sample demographics including race, ethnicity, and gender for Blueprints-certified studies:
- The Study 1 sample was 45% Black (45%), 45% Hispanic (45%), and 47% female.
- The Study 2 sample was 44% black and 47% Hispanic.
Source: Washington State Institute for Public Policy
All benefit-cost ratios are the most recent estimates published by The Washington State Institute for Public Policy for Blueprint programs implemented in Washington State. These ratios are based on a) meta-analysis estimates of effect size and b) monetized benefits and calculated costs for programs as delivered in the State of Washington. Caution is recommended in applying these estimates of the benefit-cost ratio to any other state or local area. They are provided as an illustration of the benefit-cost ratio found in one specific state. When feasible, local costs and monetized benefits should be used to calculate expected local benefit-cost ratios. The formula for this calculation can be found on the WSIPP website.
No information is available
No information is available
Program Developer/Owner
Rebecca UntermanSenior Associate, K-12 EducationManpower Demonstration Research Corporation (MDRC)11965 Venice Blvd. #402Los Angeles, CA 90066U.S.A.Rebecca.Unterman@MDRC.org
Program Outcomes
- Dropout/High School Graduation
Program Specifics
Program Type
- School - Environmental Strategies
Program Setting
- School
Continuum of Intervention
- Universal Prevention
Program Goals
A high school reform model that is academically non-selective but provides academically rigorous instruction in small, personalized learning environments Along with smaller size, the model emphasizes real-world relevance and personalized relationships between teachers and students.
Population Demographics
The program is offered in New York City and Chicago communities with schools typically having low graduation rates and low-income Black and Hispanic high school students.
Target Population
Age
- Late Adolescence (15-18) - High School
Gender
- Both
Gender Specific Findings
- Male
- Female
Race/Ethnicity
- All
Race/Ethnicity Specific Findings
- White
- Hispanic or Latino
- African American
Subgroup Analysis Details
Subgroup differences in program effects by race, ethnicity, or gender (coded in binary terms as male/female) or program effects for a sample of a specific racial, ethnic, or gender group:
- Study 1 (Bloom & Unterman, 2012, 2013) tested for subgroup differences in program effects by race, ethnicity, and gender and found equal benefits across subgroups. Tests for within-subgroup program effects found significant benefits for Blacks and Whites, Hispanics, and non-Hispanics, and males and females. In addition, Study 1 found subgroup effects by using a homogenous sample with 75% or more of students being eligible for free and reduced-price lunches.
- Study 2 (Abdulkadiroglu et al., 2013) tested for within-subgroup program effects and found significant benefits for African Americans, Hispanics, males, females, and economically disadvantaged students eligible for free and reduced-price lunches but did not report tests that compared the subgroups to one another.
Sample demographics including race, ethnicity, and gender for Blueprints-certified studies:
- The Study 1 sample was 45% Black (45%), 45% Hispanic (45%), and 47% female.
- The Study 2 sample was 44% black and 47% Hispanic.
Other Risk and Protective Factors
School
-Academic Rigor (Not measured)
-Real World Relevance (Not measured)
-Personalized Relationships (Not measured)
Risk/Protective Factor Domain
- Individual
- School
Risk/Protective Factors
Risk Factors
Protective Factors
School: Opportunities for prosocial involvement in education
*Risk/Protective Factor was significantly impacted by the program
Brief Description of the Program
New York City's Small Schools of Choice (SSCs) are academically non-selective but provide academically rigorous instruction in small, personalized learning environments for low-income high school students. Classes are generally smaller than those in public high schools, with more individualized attention given to students so that personal relationships are developed between teachers and students. In addition, the schools partner with outside organizations to assist with hiring exceptional teachers and staff as well as to provide students with opportunities to connect what they learn in school to real-world applications. Other implementations of the program such as in Chicago emphasize the small number of students, with schools having flexibility in choice of themes and curricula.
Description of the Program
New York City's Small Schools of Choice (SSCs) are teacher, parent, and community-organized schools which are academically non-selective but designed to provide academically rigorous instruction in small, personalized learning environments for low-income students. Classes are generally smaller than those in public high schools, with more individualized attention given to students so that personal relationships are developed between teachers and students. In addition, the schools partner with outside organizations to assist with hiring exceptional teachers and staff as well as providing students with opportunities to connect what they learn in school to real-world applications.
In addition to small size and location mainly in disadvantaged neighborhoods, SSCs have other defining traits:
- All are created through a competitive proposal process in which teachers, parents, and community members come forward and form school planning committees.
- Most orient at least a portion of their school missions and curricula toward a specific academic, artistic, social justice, or professional theme.
- Most are founded in partnership with a local nonprofit organization or private employers that offer students learning opportunities in and out of the classroom, and additional support staff and resources.
- Almost all are provided additional philanthropic funds during their first four or five years of startup.
- The Department of Education offers exemptions from having to enroll special education students and English language learners during the two-year startup period.
- Almost all are started with the support of an intermediary school partner.
Theoretical Rationale
The closure of several large high schools serving low-income communities in New York City served as the catalyst for Small Schools of Choice. The schools are based on the idea that personalized relationships between students and staff result in greater student learning and retention, ultimately increasing high school graduation, college enrollment, and future academic success.
Theoretical Orientation
- Situational
- Person - Environment
Brief Evaluation Methodology
Primary Evidence Base for Certification
Of the four studies Blueprints has reviewed, two studies (Studies 1 and 2) meet Blueprints evidentiary standards (specificity, evaluation quality, impact, dissemination readiness). The studies were conducted by independent evaluators.
Study 1
Bloom and Unterman (2013) used a lottery-based quasi-experimental design, with a lottery partially determining whether ninth-grade students would attend the small schools or standard high schools. The sample had 12,130 lottery students. The outcomes included test scores, graduation, and college attendance.
Study 2
Abdulkadiroglu et al. (2013) used a lottery-based quasi-experimental design, with a lottery partially determining whether ninth-grade students would attend the small schools or standard high schools. The sample had 10,204 lottery students. The outcomes included test scores, graduation, and college attendance.
Outcomes (Brief, over all studies)
Primary Evidence Base for Certification
Study 1
Bloom and Unterman (2013) found that students enrolled in New York City's Small Schools of Choice compared to similar students enrolled in other public schools were significantly more likely to graduate from high school on time and demonstrate college readiness in English.
Study 2
Abdulkadiroglu et al. (2013) found that students enrolled in New York City's Small Schools of Choice compared to similar students enrolled in other public schools were significantly more likely to graduate from high school on time, have high Regents test scores, obtain more high school credits, stay on grade schedule, and enroll in postsecondary education.
Outcomes
Primary Evidence Base for Certification
Study 1
Bloom and Unterman (2013) found that students enrolled in Small Schools of Choice in New York City were significantly more likely than similar students in other schools to
- Graduate high school
- Demonstrate college readiness in English.
Study 2
Abdulkadiroglu et al. (2013) found that students enrolled in Small Schools of Choice in New York City were significantly more likely than similar students in other schools to
- Perform better on Regents Exams in several fields
- Graduate high school on time
- Obtain more high school credits
- Stay on grade schedule
- Enroll in postsecondary education.
Generalizability
Two studies meet Blueprints standards for high-quality methods with strong evidence of program impact (i.e., "certified" by Blueprints): Study 1 (Bloom & Unterman, 2013) and Study 2 (Abdulkadiroglu et al., 2013). The samples for the studies included high school students living in disadvantaged areas of large cities.
- Study 1 took place in New York City and compared the treatment group of students attending small schools to the control group of similar students attending larger schools.
- Study 2 took place in New York City and compared the treatment group of students attending small schools to the control group of similar students attending larger schools.
Potential Limitations
Additional Studies (not certified by Blueprints)
Study 3 (Schwartz et al., 2013)
- Non-random assignment but with adjustments using an instrumental variable measuring nearness to a small school
- Many significant differences between conditions at pretest (though adjusted for with the instrumental variable)
- Weak or iatrogenic effects for old small schools
Schwartz, A. E., Stiefel, L., & Wiswall, M. (2013). Do small schools improve performance in large, urban districts? Causal evidence from New York City. Journal of Urban Economics, 77, 27-40.
Study 4 (Barrow et al., 2014)
- Non-random assignment but with adjustments using an instrumental variable measuring nearness to a small school
- Many significant differences between conditions at pretest (though adjusted for with the instrumental variable)
- Differential attrition but with imputed missing data
- One possible iatrogenic effect
Barrow, L., Schanzenbach, D. W., & Claessen, A. (2014). The impact of Chicago's small high school initiative.Federal Reserve Bank of Chicago, WP 2014-20.
Notes
Two earlier studies (Bloom, Thompson, & Unterman, 2010; Bloom & Unterman, 2012) reported on the program's impact for the first two student cohorts, which were similar to those reported in the evaluation of three cohorts in Study 1 (Bloom & Unterman, 2013). Also, in a policy brief with few details, Unterman (2014) reported on postsecondary outcomes using four cohorts.
Bloom, H. S., & Unterman, R. (2012). Sustained positive effects on graduation rates produced by New York City's small public high schools of choice. New York: MDRC.
Bloom, H. S., Thompson, S. L., & Unterman, R. (2010). Transforming the high school experience: How New York City's new small schools are boosting student achievement and graduation rates. New York: MDRC.
Endorsements
Blueprints: Promising
Social Programs that Work:Near Top Tier
Program Information Contact
Rebecca Unterman
Senior Associate, K-12 Education
Manpower Demonstration Research Corporation (MDRC)
11965 Venice Blvd. #402
Los Angeles, CA 90066
Rebecca.Unterman@MDRC.org
References
Study 1
Bloom, H. S., & Unterman, R. (2014). Can small high schools of choice improve educational prospects for disadvantaged students? Journal of Policy Analysis and Management, 33(2), 290-319.
Certified Bloom, H. S., & Unterman, R. (2013). Sustained progress: New findings about the effectiveness and operation of small public high schools of choice in New York City. New York: MDRC.
Certified Unterman, R. (2014). Headed to college: The effects of New York City's small high schools of choice on postsecondary enrollment. New York: MDRC.
Study 2
Certified Abdulkadiroglu, A., Hu, W., Pathak, P. A. (2013). Small high schools and student achievement: Lottery-based evidence from New York City. NBER Working Paper No. 19576.
Study 3
Schwartz, A. E., Stiefel, L., & Wiswall, M. (2013). Do small schools improve performance in large, urban districts? Causal evidence from New York City. Journal of Urban Economics, 77, 27-40.
Study 4
Barrow, L., Schanzenbach, D. W., & Claessen, A. (2014). The impact of Chicago's small high school initiative. Federal Reserve Bank of Chicago, WP 2014-20.
Study 1
Summary
Bloom and Unterman (2013) used a lottery-based quasi-experimental design, with a lottery partially determining whether ninth-grade students would attend the small schools or standard high schools. The sample had 12,130 lottery students. The outcomes included test scores, graduation, and college attendance.
Bloom and Unterman (2013) found that students enrolled in Small Schools of Choice in New York City were significantly more likely than similar students enrolled in other schools to:
- Graduate high school
- Demonstrate college readiness in English.
Evaluation Methodology
Design:
Recruitment: The sample included first-time ninth-graders in New York City who entered high school in the fall of 2005, 2006, or 2007. Exclusion criteria included attending a parochial or private school in eighth grade, and missing scores on New York state eighth-grade reading and math tests. Because data were collected and analyzed retrospectively, the researchers did not directly recruit participants.
Assignment: The study used a quasi-experimental, instrumental variable design based on both the actual participation in the program and an instrument measuring randomized assignment into the program.
First, randomization was used for initial assignment. Group assignment was based on the order in which students were listed in the New York City High School Application Processing System during their first high school lottery (of multiple possible lotteries they might enter during the high school matching process). High school assignment was based on a student-school matching process in which students rank-ordered up to 12 school preferences and schools submitted geographic and other constraints for their students. When schools became oversubscribed, students were submitted to a random lottery and assigned based on their random order in the assignment queue. The authors claimed this lottery system ensured that student rank-ordered preferences for a treatment school did not influence whether they were assigned to it, though students only entered a lottery for a treatment school if the treatment school appeared on their list of their top 12 choices.
Second, actual participation or non-participation in the program defined the two conditions. The intervention group included students attending Small Schools of Choice who ranked their schools as their first choice in the lottery and were involved in a lottery resulting in their random assignment to that school. The control group included students not attending Small Schools of Choice who either did not rank Small Schools of Choice in their top choices or did not get assigned to their choice of treatment school. Each lottery for each individual Small School of Choice produced an intervention group who won the lottery and enrolled in the school, and a control group who lost the lottery and then enrolled in another school that was not a Small School of Choice. The randomized sample included lotteries for 199 schools, for 84 SSCs and a total of 14,969 students.
Attrition: The four-year follow-up sample consisted of 12,130 students (81%). Attrition included 2,553 students without four-year follow-up data and 286 students in lotteries that became incomplete due the exclusion of the students with missing follow-up data. Reasons for attrition included not staying in or graduating from high school. Estimated effects persisted but decreased in magnitude across two sets of analyses using different approaches to impute missing follow-up data.
Sample: The treatment schools were all opened since 2002, contained fewer than 550 students across all grades, and served some of the district's most disadvantaged communities. Because the schools were academically non-selective, they had students from varying academic backgrounds.
The students in the evaluation tended to be Black (45%) or Hispanic (45%) and from low-income communities (84% eligible for free or reduced-price lunch). Slightly fewer boys (47%) enrolled than girls, and very few were English language learners (7%) or enrolled in special education (6%).
Measures: All study outcomes were taken from New York City Department of Education administrative records or other existing data sources. Student measures included eligibility for free or reduced-price lunch, race/ethnicity, gender, and 8th grade reading proficiency based on a standardized test.
On-Time Graduation equaled graduation by the end of the student's fourth year of high school. Three types of diplomas were offered, each with different standards reflecting the students' college readiness: 1) Local Diplomas with the least stringent requirements, which were phased out over the course of the study; 2) Regents Diplomas considered to be the standard credential, requiring students to pass all required courses and score at least 65/100 on each of five Regents examinations covering English Language Arts, Mathematics, Science, Global History, and American History; and 3) Advanced Regents Diplomas with the most stringent requirements for graduation.
College Readiness was based on students' Regents examination scores (in English and Math), using a threshold of 75 defined by the City University of New York as high enough to exempt incoming college students from having to take remedial English or Math courses.
Analysis: The researchers used an instrumental variables approach to estimate the average effect of enrolling in a treatment school for students who did so because they won their first high school assignment lottery, which is a local average treatment effect. The instrument was a student's "random" assignment from their first high school assignment lottery, which was used to predict enrollment in a treatment school, which was in turn used to estimate the causal effect of the treatment on the academic outcome variables of interest. School-specific lotteries were treated as blocks, and eighth grade reading and math test scores were included as baseline outcome covariates. All models employed two-stage least square estimation to account for clustering of students within schools.
Using the same analytic strategy, subgroup analyses examined whether program impacts differed by cohort, 8th-grade reading and math proficiency, free/reduced-price lunch eligibility, race/ethnicity, gender, special education and English language learner status, and other lottery-related variables.
Intent-to-Treat: Consistent with an intent-to-treat approach, the study used all available data and replaced missing data using both an informed, model-based imputation, and a conservative method, assuming that all missing students failed to graduate. Also, the study excluded students in lotteries who were assigned to schools that did not open or were involved with lotteries that had zero compliance. Otherwise, the instrumental variable approach may violate the intent-to-treat criterion by examining the effects of actual participation in the program rather than the effects of random assignment to the program. However, unlike a treatment-of-the treated analysis, the instrumental variable approach minimizes rather than increases selection bias. The authors note that Table B1 shows similar effects when testing for the effect of original assignment.
Outcomes
Implementation Fidelity: There were no strict guidelines of adherence for the SSCs, given that the idea behind the schools is for teams of teachers, administrators, and community leaders to structure the schools how they see fit to promote academic rigor and personal relationships. Therefore, the only quantitative measures of fidelity referred to the size of schools in the two conditions.
Baseline Equivalence: The study did not compare the conditions directly but focused on the randomized lottery groups. There were no statistically significant differences on baseline characteristics between students winning and not winning the lottery, though there were marginally (p< .07) more control students identifying as (see Table A.1 in Bloom & Unterman, 2013).
Differential Attrition: Students missing graduation data were more likely to have entered high school over-age for their grade level and less likely to be on track toward graduation in 9th grade. However, tests comparing the characteristics of lottery winners and control groups students who were retained for the duration of the study and were included in the analytic sample revealed no significant differences (see Table A.2 in Bloom & Unterman, 2013). Also, use of two imputation procedures maintained the direction and significance of the intervention effects.
Posttest: At the four-year follow-up, enrolling in a treatment school was associated with a significant estimated increase in the four-year graduation rate, and this finding held across all three treatment cohorts, indicating the treatment effect replicated for three annual years. Specifically, the increase in graduation was attributable to treatment group students being significantly more likely to receive a Regents diploma than other students. Similarly, data from the first two cohorts indicated that the five-year graduation rate was higher for students enrolled in treatment schools as compared to students in control schools. Also, treatment students shows increased college-readiness on the state standardized reading examination (but not the math examination).
Subgroup analyses showed that the increase in four-year graduation rates were significantly moderated by eighth-grade reading and math proficiency scores, such that the effects occurred for students scoring below or at their grade level in their eighth-grade reading and math proficiency scores, but not for those scoring above their grade levels.
Study 2
Summary
Abdulkadiroglu et al. (2013) used a lottery-based quasi-experimental design, with a lottery partially determining whether ninth-grade students would attend the small schools or standard high schools. The sample had 10,204 lottery students. The outcomes included test scores, graduation, and college attendance.
Abdulkadiroglu et al. (2013) found that students enrolled in Small Schools of Choice in New York City were significantly more likely than students enrolled in other schools to
- Perform better on Regents Exams in several fields
- Graduate high school
- Obtain more high school credits
- Stay on grade schedule
- Enroll in postsecondary education.
Evaluation Methodology
Design:
Recruitment: The study identified 151 eligible Small Schools of Choice (SSC) programs in New York City. It then used 108 of those schools that met the criteria for inclusion in the lottery sample: The school must have been oversubscribed for at least one year between application years 2003-04 and 2007-08, and it must have had a set of applicants who were subject to a lottery in New York City's high school match. The sample of schools formed after 2002 and oversubscribed for some of their seats was generally newer and more popular than other SSCs.
The analysis sample included students who were enrolled in a New York City school and applied to SSCs in one of the five school years from 2003-04 through 2007-08 for fall 9th grade enrollment. Students within the 108 schools were selected for the study if 1) they ranked an SSC as their top choice and 2) were subject to a lottery. A total of 10,204 students met both criteria (Table 2, column 7). The study excluded students who 1) did not rank an SSC on their preference list, 2) ranked an SSC later on their preference list but not first, and 3) ranked an SSC as first on their preference list but were assigned to one where there were enough spaces for the applicants to avoid a lottery.
Assignment: The study used a quasi-experimental, instrumental variable design based on both the actual participation in the program and an instrument measuring randomized assignment into the program.
First, randomization was used for initial assignment. Assignment to schools under the New York City system was based on a combination of student preferences, school priority, and random assignment. Student applicants ranked their school preferences and schools assigned priority to students. The assignment to a school often depended on winning and losing the lottery among students with the same preference and priority. Students who won the first lottery in which they participated and received an offer to attend their preferred SSC defined one group (coded 1) and students who did not win the first lottery defined another group (coded 0). This randomized grouping was used as an instrumental variable. A complication came from students who lost the first lottery for their highest preferred school but won a lottery for their second or third preferred SSC. Although these students likely received offers and attended an SSC, the study treated them according to the original grouping based on the first lottery. The instrument was coded as zero in this case, since the student did not obtain an offer at the first choice school.
Second, actual participation or non-participation in the program defined the two conditions. Since lottery losers could still attend an SSC, the randomization did not define condition participation. The study used actual years attended and attendance in 9th grades for the conditions. The authors noted (p. 10) that, despite the use of a lottery, student school choices and enrollment in an SSC depended on more than random assignment and "may be related to unobserved variables such as family characteristics and motivation." The analysis adjusted for this bias with the instrumental variable strategy.
Attrition: The authors state that over 70% of students took the Regents Math exam, and around 65% took the other Regents exams (p. 18). As shown in Tables 5-7, the sample sizes and attrition varied substantially across the other outcomes.
Sample Characteristics: Table 2 presents student characteristics for a variety of populations but column 6 on lottery applicants seems most relevant. The students were 44% black and 47% Hispanic, with 71% eligible for a subsidized lunch. The lottery sample had no students in special education or having limited English-language proficiency. Baseline math and reading test scores were below the city average. Overall, the lottery sample appeared somewhat more disadvantaged than all city students, and possibly differing in some ways from all those attending SSCs.
Measures: The program measure of years enrolled in an SSC from 2002-03 through 2009-10 came from New York City registration information. Baseline (i.e., pre-treatment) test scores were based on 8th grade standardized exams in Math and English Language Arts and also came from New York City records.
Regents test scores came from a mandatory state examination used to determine if a student was eligible for a high school diploma in New York. The scores were standardized to the city distribution of scores by grade, test date, year, and subject. There is some concern that some schools selectively re-graded the exams to improve scores.
Other outcomes obtained from city records included: credits, grade progression, attendance, high school graduation, preliminary SAT (PSAT) test scores, SAT Reasoning test scores, and Advanced Placement (AP) test scores. Data on college enrollment came from the National Student Clearinghouse, as reported to the city. Different outcomes were reported at different time points for the students (see Table B3).
Analysis: To assess program effects, the study examined the influence of the years spent in an SSC as of the date of test score outcome measures. For outcomes such as graduation and college attendance, the study examined the influence of whether a student attended an SSC in 9th grade (which avoids problems of reverse causality in which graduation affects the time spent in a program school).
Model estimation used an instrumental variable that measured whether or not the subject won the first lottery for a preferred SSC. The strategy exploited the partial random assignment of students to schools and adjusted for unobserved variables such as family characteristics and motivation that influence the student choice of schools. As stated on page 11, the model estimated the causal effect of the program for those who received an offer to attend a SSC and complied with the offer by enrolling in an SSC. The model estimates also clustered standard errors by school-test year for test outcomes and by student for repeated measure outcomes such as credits and attendance. Controls included 8th grade test scores, demographic covariates, fixed effects for year of test and grade of test, and dummy variables for "lottery specific risk sets."
Intent-to-Treat: The study used all available cases with posttest data, and checked results for the full sample after imputing missing posttest data. It included SSCs that were later closed because of poor performance (p. 26). Otherwise, the instrumental variable approach may violate the intent-to-treat criterion by examining the effects of actual participation in the program rather than the effects of random assignment to the program. However, unlike a treatment-of-the treated analysis, the instrumental variable approach minimizes rather than increases selection bias.
Outcomes
Implementation Fidelity: Students who both won the lottery and attended SSCs on average had just over 161 9th grade classmates and 443 students across all high school grades. That compares with 715 9th grade classmates and 2,117 schoolmates for other schools. Also, applicants spent 0.46 years longer in an SSC before taking the Regents Math exam if they were randomly offered a seat than those who were not. The time spent in an SSC was .76 years longer before the English Regents exam. Surveys generally indicated that the SSC environment was associated with higher levels of engagement, greater safety and respect, higher academic expectations, and better communication (p. 30). Teacher surveys affirmed the student-reported differences between SSCs and others (p. 31). Table 3 and pages 16-17 identify some additional differences between the school environments experienced by lottery winners who attended an SSC and lottery losers who did not attend an SSC.
Baseline Equivalence: The randomized lottery appears to have worked well. Table 2 (columns 7-8) shows differences on demographic characteristics and baseline scores between lottery participants who were offered and not offered a seat in an SSC. The reported differences were regression-adjusted for risk-set dummy variable controls. The differences were small and none were statistically significant. A joint test for all variables also showed no significant difference.
Differential Attrition: Page 19 and Table A3 indicate that lottery winners were more likely than non-winners to take several of the Regents exams and have lower attrition. A check for the influence of this attrition used the 8th grade baseline test scores to replace the missing posttest outcomes. The results for the full sample with imputed outcomes were somewhat weaker but similar to results for the sample with complete data.
Outcomes
Posttest: The two-stage least squares estimates with the lottery outcome as an instrumental variable show the adjusted effect of an additional year spent enrolled in an SSC.
Regents Tests: The additional year significantly increased the Regents test scores (Table 4) on four of five subjects: by 0.14 standard deviations for Math, 0.11 standard deviations for English, 0.18 standard deviations for Living Environment, and 0.09 standard deviations for US History. The effect on the English exam dropped to insignificance after adding controls for demographic characteristics and baseline scores (Table 4) and after dropping schools that were audited for troubling patterns of test scores (Table A6). Tests for the effect of school size per se (Table 12) suggested that dramatic rather than incremental reductions in school size are needed to improve test scores.
Other Outcomes: Attending an SSC significantly improved school credit accumulation, starting grade on time, and attendance (Table 5), receipt of a high school diploma and scoring high enough on the Regents exam to not need reading or writing remediation (Table 6), and the likelihood of taking the PSAT exam but not the SAT or advanced placement exams (Table A4).
Subgroup Analyses: Comparisons of enrollment effects by gender, race/ethnicity, baseline test scores, period of opening, and intermediary organization generally showed program benefits across all groups, including more disadvantaged minority and low SES groups. However, students with baseline scores above the median showed more consistent benefits of program enrollment.
Long-Term: The measures for ever having attended college and attending for 2-4 semesters (i.e., college persistence) assessed outcomes after leaving high school and likely at least a year after the program ends. The results showed that an additional year of enrollment in an SSC significantly increased six of eight measures of college enrollment, but effects on two measures of college persistence were only marginally significant.
Study 3
Summary
Schwartz et al. (2013) used a quasi-experimental design based on the nearness of the student's location to a small school. The study had a large sample and examined dropping out, graduation, and test scores.
Schwartz et al. (2013) found that attending small schools compared to similar students attending other schools had significantly
- Higher on-time graduation rates but only for newer small schools established since 2002.
Evaluation Methodology
Design:
Recruitment: The study included cohorts of students expected to graduate in either 2007 or 2008 and all high schools attended by the cohorts (excepting specialized program schools such as last chance high schools or schools for pregnant mothers and schools with predominantly full-time special education students). It excluded schools and students located on Staten Island because no small high schools existed there and students generally did not travel elsewhere to attend small schools. The full sample had 291 schools and 76,213 students.
Assignment: The study used a quasi-experimental, instrumental variable design based on both the actual participation in the program and an instrument measuring the distance between the nearest small school or large school and the student's home.
First, actual participation or non-participation in the program defined the two conditions. Students were non-randomly assigned in the study to the school in which they enrolled in 9th grade, and were treated in the original assignment group even if they transferred later. School size equaled the total number of students enrolled at each high school in a student's 9th grade year, with a small school having 550 or fewer students. There were 169 small and 122 large schools with 15,444 and 60,769 students, respectively. A new school was defined as one that had no graduates in the 2001 or 2002 cohorts but did have graduates in the 2007 or 2008 cohorts.
Second, a measure of the distance between the nearest small school or large school and the student's home defined an instrumental variable that was used to adjust the actual program participation for selection bias. The authors argue that the measure exogenously influences student decisions to attend small schools but does not influence student outcomes.
Attrition. The sample included those enrolled in both NYC public middle schools in 8th grade and public high schools in 9th grade and excluded students who entered or left the NYC public system between middle and high school. No figures were available on exits and entrances.
Sample Characteristics: The sample appeared disadvantaged, with mostly black and Hispanic students, less than 60% speaking English as a home language, and 75% eligible for free or reduced-price lunch. The small school sample subjects were generally more disadvantaged than the large school sample.
Measures: The measures came from NYC Department of Education administrative datasets. The student data included sociodemographic characteristics and academic measures such as being an English language learner, being over-age for grade, and prior test scores in 8th grade reading and mathematics (converted to z scores with mean zero and standard deviation of one for each sample cohort). Residence zip codes and school addresses in the data enabled calculation of distance, in miles, between home and schools.
The outcomes included graduation, defined as earning a diploma in four years, and Regents exam scores in English and mathematics. The measures equaled whether students took these exams, and conditional on taking them, whether their scores met the cutoffs for various kinds of diplomas.
Analysis: Because students attending small schools were more disadvantaged and possibly more motivated to improve their school performance, comparisons across schools could be biased. The instrumental variable approach to deal with this bias first predicted small school attendance using quadratics for distance to nearest small school and distance to nearest large school as instruments. The distance to the nearest small school significantly reduced enrollment (and, as shown by the quadratic, the negative effect leveled off at longer distances). The model then used predicted small school enrollment as a determinant of the outcomes. The outcome models used linear regression with borough fixed effects, and standard errors were adjusted for heteroskedasticity and clustering of students at the school level. Baseline controls included 8th grade tests scores and a variety of sociodemographic variables.
Tests for heterogeneity included separate indicators for old and new small schools, which were modeled separately in the instrumental variable approach but used together in the outcome model.
Intent-to-Treat: Although students were able to transfer among high schools, and about 15-20% changed high schools, subjects were assigned to their 9th grade school in order to obtain an intent-to-treat estimate. Otherwise, the instrumental variable approach may violate the intent-to-treat criterion by adjusting the conditions for distance from the school rather than using the conditions alone. However, unlike a treatment-of-the treated analysis, the instrumental variable approach minimizes rather than increases selection bias.
Outcomes
Implementation Fidelity: Since the key program component was the number of students, the study presented no information on implementation fidelity.
Baseline Equivalence: Given that students largely self-selected into small and large schools, the two conditions were not equivalent. Table 1 shows that the small schools had a more disadvantaged student population on eligibility for free or reduced-price lunches, over-age for grade, and 8th grade test scores. The one exception was that small school students had a higher proportion with English as a home language.
More important for comparisons across conditions, the instrumental variable approach assumes that distance to the nearest large and small schools was unrelated to outcomes. Several tests helped check this assumption. First, new small schools might be located in areas with high demand for better schools and more motivated students. However, analysis (p. 34) indicated "that new small schools were not placed strategically with respect to outcomes of students in earlier cohorts." Second, families of motivated students might move to be closer to small schools. Analysis (p. 34) suggested that previous moves across zip codes were unrelated to the location of old and new small high schools. Third, motivated students not living near small schools may leave the public school system. The sample included students who were also enrolled in middle school, thereby excluding those who entered or left the system between middle and high school. The authors emphasize that they examined program efficacy only for this particular sample. Fourth, distance to a small school might affect outcomes of students not attending that school, but the authors argue these spillover effects were relatively small (p. 34).
Differential Attrition: No attrition for this sample.
Posttest: Although small school enrollment in the instrumental variable estimation did not significantly affect graduation, additional tests showed a positive effect of new small schools and a negative significant effect of old small schools. Because they were more likely to graduate, new small school students were significantly less likely to receive a GED.
For the other outcomes, the instrumental variable estimates showed that new small school enrollment significantly increased the probability of taking the English and Math Regents exams. However, new small school enrollment significantly reduced English test scores, perhaps because a higher proportion of the new small school students take the exam. Old small schools showed significantly worse performance on 8 of 9 outcome measures.
For subgroup analyses, new small school enrollment increased graduation for all groups, but the effect was significant (.05) for Asians, Hispanics, and the non-poor. Old small school enrollment significantly reduced graduation for 6 of the 8 groups.
Long-Term: The subjects weren't followed past high school.
Study 4
Summary
Barrow et al. (2014), used a quasi-experimental design based on the nearness of the student's location to a small school. The study had a large sample and examined dropping out, graduation, and test scores.
Barrow et al. (2014) found that students attending small schools compared to similar students attending other schools had significantly
- Lower dropout rates
- Higher graduate rates
- Better on-grade schedules.
Evaluation Methodology
Design:
Recruitment: The study used data from five cohorts of students who entered 9th grade in a Chicago public school between fall 2002 and fall 2006 and who were enrolled in an 8th grade Chicago public school during the spring of the previous school year. Table 1 shows 7,252 small school students (intervention group) and 56,731 former classmates (the control group) that meet the criteria. The total sample size thus equals 63,983.
Assignment: The study used a quasi-experimental, instrumental variable design based on both the actual participation in the program and an instrument measuring the distance between the nearest small school or large school and the student's home.
First, actual participation or non-participation in the program defined the two conditions. Students were non-randomly selected into one of the 22 small high schools or into other public high schools in Chicago. Given the non-random assignment, the groups differed substantially at pretest. Specifically, small school students entered high school with more academic disadvantages: they were more likely to have an Individualized Education Plan, be old for their grade (a proxy for being held back), have changed schools during the 8th grade school year, and score markedly worse in both math and reading exams in 8th grade.
Second, a measure of the distance between the nearest small school or large school and the student's home defined an instrumental variable that was used to adjust the actual program participation for selection bias. The authors noted that proximity to the nearest small school was a strong predictor of small school attendance but appears to be unrelated to the outcomes.
Attrition: The study followed students for 5 years after entering high school. Measures of dropping out and grade persistence have no attrition, as missing data indicated students had left school. For test scores, the study reported that, among the sample of students with baseline 8th grade test scores, 87% had 9th grade test scores, 69% had 10th grade test scores, and roughly 42% had 11th grade test scores.
Sample Characteristics: About 80 percent of the small school students were African American, 20 percent were Hispanic, and nearly 90 percent were eligible for free or reduced price lunch. Roughly one-third of the small school students were old for their grade, and almost one-quarter had some type of disability identified by having an Individualized Education Program plan. The average 8th grade math score among small school enrollees was 0.45 standard deviations below the district average and the average reading score was .34 standard deviations below the district average.
Measures: The data came from the Consortium on Chicago School Research's longitudinal dataset on student enrollment patterns and test scores. The primary outcome measures used fall administrative enrollment records to construct indicators of whether a student was still enrolled, was progressing from grade to grade on time, and graduated from high school. The raw data distinguished between dropouts and transfers to parochial or suburban schools, but the authors note that there might be incentives to erroneously code a student as a transfer instead of a dropout, particularly in small schools. Therefore, the study combined leavers and dropouts in measures of dropping out, grade progression, and graduation.
The five dropout measures were defined as leaving in years 1-5, the three persistence measures as being on time in grades 10-12, and the two graduation measures as graduation on time and graduation in 5 years.
The standardized test score outcomes came from exams given to students in the fall of 9th and 10th grades, and spring of 11th grade. These included EXPLORE math and reading scores from the fall of 9th grade, PLAN math and reading scores from the fall of 10th grade, and ACT math, reading, English, and science scores from the spring of 11th grade. Given substantial attrition on these variables, the study imputed missing posttest scores. The imputation assigned the percentile ranking on the most recent standardized test score available to the missing percentile ranking on the three outcome test scores. The ACT science test used the most recent math percentile ranking, and the ACT English test used the most recent reading percentile ranking.
Analysis: The tables present ordinary least squares and instrumental variable estimates of the effect of small school enrollment on the outcomes. The first stage of the instrumental variable estimation treated small school enrollment as the outcome and used a large number of controls, including 8th grade tests scores, plus an instrumental variable equal to the distance to the nearest small school. The second stage used predicted small school enrollment as the key determinant of the outcomes. The models clustered standard errors by cohort and 9th grade school.
The instrumental variable of the distance between a student's home and the nearest small school was assumed to 1) affect enrollment in a small school and 2) be unrelated to other characteristics that directly impact student outcomes. Under the assumptions, the instrumental variable approach estimated the local average treatment effect, or the causal impact of small schools on those students who decide to enroll in a small school due to its proximity.
Intent-to-Treat: The study examined all subjects with data and imputed data for those missing ACT test scores. Otherwise, the instrumental variable approach may violate the intent-to-treat criterion by adjusting the conditions for distance from the school rather than using the conditions alone. However, unlike a treatment-of-the treated analysis, the instrumental variable approach minimizes rather than increases selection bias.
Outcomes
Implementation Fidelity: The small schools on average enrolled 154 students per cohort, compared with 519 for the large high schools attended by their former classmates. No other characteristics specific to the program besides small size are relevant to implementation fidelity.
Baseline Equivalence: Table 1 shows numerous differences on demographics and prior test scores between those attending small schools in 9th grade and their 8th grade classmates who attended other public high schools. In support of the instrumental variable approach, proximity strongly predicted small school attendance (Table 3). Also in support, proximity had insignificant relationships with 8th grade math scores, student gender, unstable enrollment in 8th grade, or disability status. However, the results did show relationships of proximity with 8th grade reading scores, free lunch status, and student race.
Differential Attrition: The study noted a possible problem from differential attrition, as missing exam scores likely related to differences in dropout rates between small high schools and other public high schools. However, rather than performing formal tests for differential attrition, the study assumed the existence of the problem and addressed it by imputing missing data.
Posttest: In the final model of Table 4 (instrumental variable estimation with fixed effects for ZIP code and neighborhood school), enrollment in a small school significantly improved 7 of 10 outcomes (p < .05). The significant effects included lower dropping out at years 2, 3 and 5, higher on-time grade at 11th and 12th grade, and graduation on time and after 5 years.
Subgroup analyses suggested benefits of small schools for all groups but somewhat stronger benefits for boys than girls, for those with high prior tests scores than those with low prior test scores, and for the learning disabled. Black students tended to benefit in the early years, while Hispanic students benefitted more in the later years.
For test scores in Table 7, no significant program effects were apparent in the instrumental variable model, but there was one marginal negative effect that became significant after imputing missing data. The negative effect suggests that small school students did worse on the 11th grade Math ACT score.
Long-Term: Students were not followed past high school and the end of participation in the small schools.